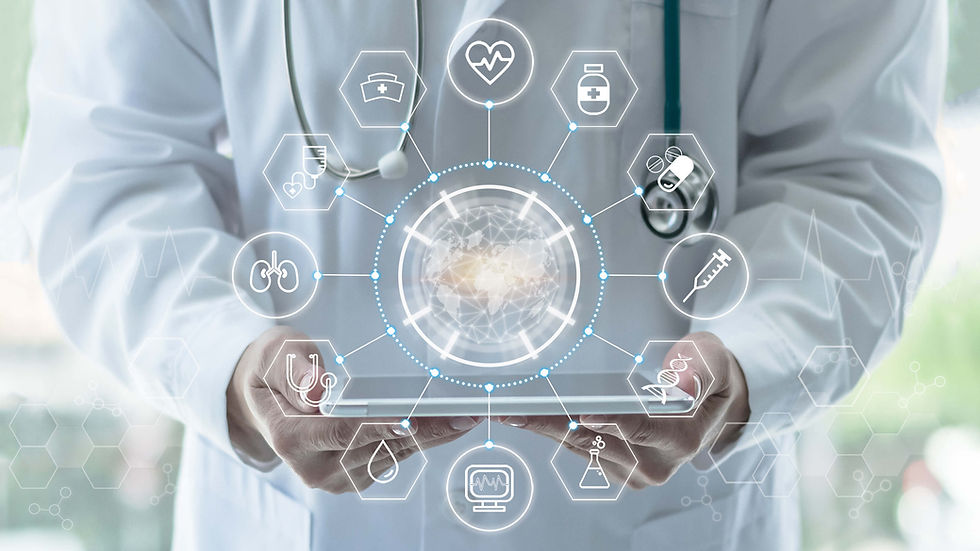
Authors: Anushka Gundluru, Annabella Irani Fey, Harini Sivanandh Ramadass, Alice Sullivan, and Aryk Tyagi
Mentor: Fiona Hartley. Fiona is currently a doctoral candidate in the Department of Oncology at the University of Oxford.
Abstract
This review paper examines the impact of big data on modern medicine, focusing on genomics, transcriptomics, metabolomics, and proteomics, which have advanced our understanding of biological processes and enabled personalized medicine. We reviewed studies on the applications of omics technologies in understanding disease and treatment, with key sections on genomics in cancer research and epidemiology. We also explore transcriptomics for gene expression analysis, metabolomics for disease biomarkers, and proteomics for cancer diagnostics and classification. Success stories include multi-omics approaches for personalized cancer treatment and improved drug response. The review also discusses the challenges of integrating multi-omics data and the need for refined analytical tools, highlighting the need for further research in optimizing multi-omics integration to produce even more personalized medicine.
Introduction
“Omics” refers to the examination of large-scale data that reflects the structure and function of a biological system at a specific level. This methodology has significantly reshaped how biological systems are studied. "Top-down" techniques, which start with general information and move towards the specific, are largely fueled by omics technologies. These work in tandem with traditional "bottom-up" approaches, creating a comprehensive framework for exploring biological systems. Consequently, the investigation of complex diseases such as cancer has progressed from a simplistic, low-throughput analysis of malignant and healthy cells to a more dynamic, spatio-temporal investigation. This approach examines intricate systems by exploring multi-layered changes across genomic, transcriptomic, proteomic, and metabolic levels in an unbiased manner (Dai & Shen, 2022).
In the late 20th century, Leroy Hood and his team developed an automated DNA sequencer and the ink-jet DNA synthesizer, the first omics technologies (History Associates Incorporated, 2021). This team also introduced the protein sequencer and protein synthesizer to study proteins. The intent was to understand complex biological systems more holistically. These high-throughput biochemical assays measure identical molecules from a biological sample. The "omics" notion refers to the fact that nearly all instances of the targeted molecular space are measured in a particular assessment, providing thorough and holistic views of a given biological system (History Associates Incorporated, 2021). Methods such as these enabled the large-scale analysis of genetic information, laying the foundation for the "genomics" field and subsequently other "omics" disciplines (Micheel et al., 2012). This marked the start of the "omics revolution," where researchers could study entire sets of biological molecules simultaneously (Oliver et al. 1992). The four main omics technologies, genomics, transcriptomics, proteomics, and metabolomics will be discussed in this review along with their application to medicine.
Literature Review
Genomics
Genomics, which is the study of the genome, involves a thorough examination of an organism's complete set of DNA. Next-generation sequencing (NGS), introduced in 2004, has largely replaced older methods like Sanger sequencing, enabling advanced analysis of the whole genome. These platforms produce large volumes of genetic data quickly and at a much lower cost. The introduction of NGS has been crucial in obtaining genome-scale data, allowing scientists to gain a better understanding of entire genomes and bridge the gap between genotype and phenotype. Genome-wide association studies (GWAS) have become the standard for identifying genetic regions in humans and other species that are associated with complex traits. This progress helps to overcome the limitations of traditional genetic association methods and enhances the understanding of unidentified and uncommon inherited diseases (Ng et al., 2010; Roach et al., 2010).
Limitations in Current Standard of Care
Genomics has changed our understanding of cancer by uncovering the DNA mutations that lead to tumor development and growth. Initiatives such as The Cancer Genome Atlas (TCGA) have identified numerous cancer-associated mutations, enabling the classification of tumors based on their genetic profiles and paving the way for precision medicine (National Cancer Institute, n.d.).
BRCA1 and BRCA2
Mutations in the BRCA1 and BRCA2 genes can lead to increased cancer susceptibility, particularly in breast and ovarian cancers. Cancer cells with BRCA-mutations are highly sensitive to poly (ADP ribose) polymerase (PARP) inhibitors because mutations in BRCA1 or BRCA2 impair the cell’s ability to repair DNA damage through homologous recombination. This makes them vulnerable to further damage caused by PARP inhibition (Rose et al., 2020; Turk & Wisinski , 2018). As PARP is essential for repairing single-strand DNA breaks, its inhibition leads to double-strand breaks that rely on BRCA-mediated repair (Dahlstrom, 2024). Without functional BRCA, these errors accumulate and result in tumor cell death, a concept known as synthetic lethality (Rose et al., 2020; Turk & Wisinski, 2018).
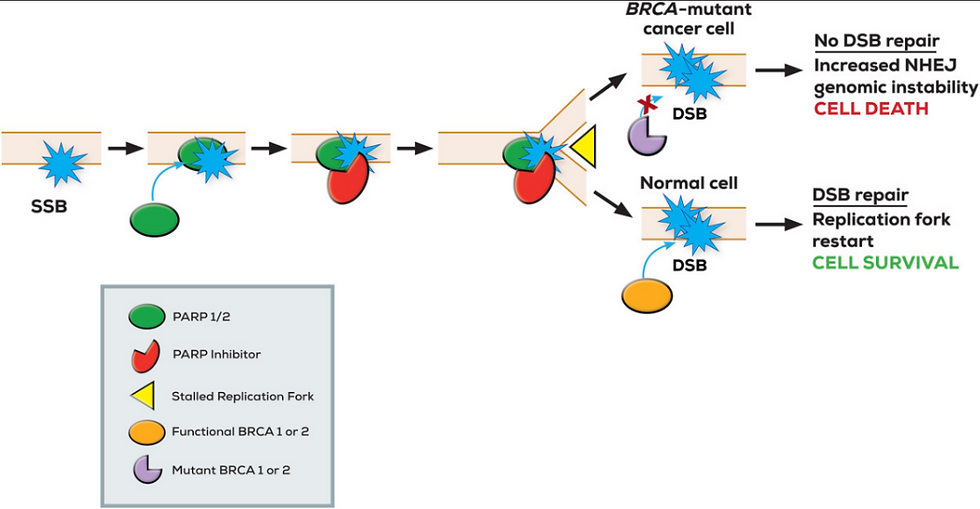
Figure 1: A single-strand DNA break (SSB) leads to poly(ADP-ribose) polymerase (PARP) activation, but PARP inhibition results in persistent SSBs and stalled replication forks, causing double-strand DNA breaks (DSBs) to occur. In BRCA-mutant cells, defective homologous recombination repair means these DSBs cannot be repaired, and this ultimately leads to cell death in a process known as synthetic lethality. Normal cells with functional BRCA repair are able to repair the damage and survive. Figure taken from Turk & Wisinski (2018).
Most breast cancers are not inherited, but about 5-10% are passed down through families, often due to changes in the BRCA1 or BRCA2 genes, which can lead to hereditary breast and ovarian cancer syndrome (Turk & Wisinski, 2018). BRCA1, found on chromosome 17, and BRCA2, located on chromosome 13, are important for fixing DNA damage. When these genes are mutated, they can dramatically increase the risk of breast cancer, with a lifetime risk as high as 84% (Turk & Wisinski, 2018). BRCA1 mutations are often linked to earlier, more aggressive forms of breast cancer, including triple-negative breast cancer, while BRCA2 mutations are more likely to lead to breast cancer that is hormone receptor-positive. Genetic testing is recommended for anyone under 60 with triple-negative breast cancer, as 15-33% may have a BRCA mutation (Turk & Wisinski, 2018).
BRCA1 and BRCA2 in the Clinic
Studies conducted by Audeh et al. (2010) and Tutt et al. (2010) demonstrated that the loss of BRCA function combined with PARP inhibition was lethal for tumor cells yet spared normal cells with intact BRCA (Hutchinson, 2010). Olaparib, an orally active PARP inhibitor, was tested in phase II studies led by Andrew Tutt and William Audeh on patients with advanced breast or ovarian cancer harboring BRCA mutations (Audeh et al., 2010; Tutt et al., 2010).
Tutt et al. (2010) separated women with advanced breast cancer and BRCA1 or BRCA2 mutations into two groups. The first cohort received a high-dose of olaparib (400 mg) twice a day and had an objective response rate (ORR) of 41% and median progression-free survival (PFS) of 5.7 months, compared to 22% ORR and 3.8 months PFS in the low-dose group (100 mg). The study demonstrated positive proof of concept for PARP inhibition in BRCA-deficient breast cancers, with a favorable therapeutic index. Adverse events, primarily low-grade fatigue, nausea, and anemia, were consistent with previous findings, supporting the efficacy and safety of this targeted treatment strategy.
The study by Audeh et al. (2010) revealed similar dose-dependent outcomes in recurrent ovarian cancer. The first cohort received oral olaparib at 400 mg twice daily, and the second cohort received 100 mg twice daily. ORR was 33% in the high-dose cohort with 5.8 months PFS, while the ORR was only 13% in the low-dose cohort and 1.9 months PFS. Findings from this study provide proof of concept for the efficacy and tolerability of genetically targeted treatment with olaparib in BRCA-mutated advanced ovarian cancer.
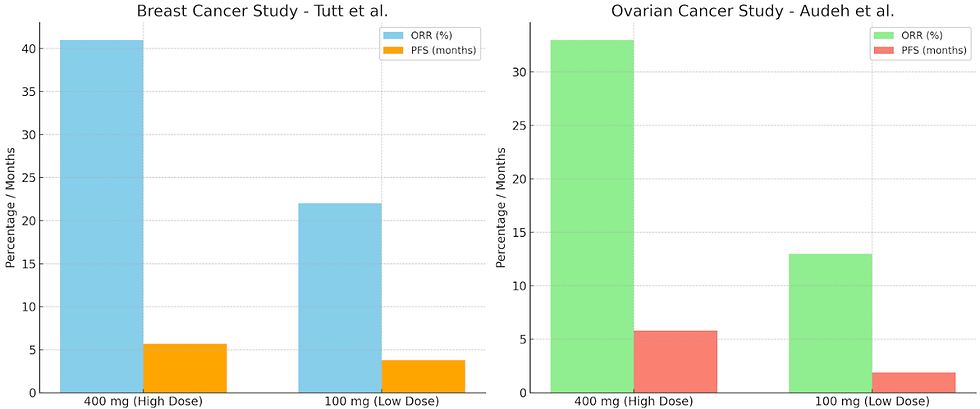
Figure 2: The objective response rate (ORR) and progression-free survival (PFS) rates from two olaparib clinical trials showing dose-dependent effects. The left panel displays results from Tutt et al. (2010) and the right panel displays results from Audeh et al. (2010).
These studies highlight the importance of targeting specific genetic abnormalities rather than focusing solely on the tumor's organ of origin. By identifying BRCA mutations, genomics enables the use of PARP inhibitors like olaparib, offering a promising therapeutic option for patients who might otherwise be resistant to standard treatments. This reinforces the importance of genomic profiling in personalizing cancer treatments and improving patient outcomes.
Genetic Counseling
Genetic counseling is a process where individuals or families receive information, guidance, and support regarding their genetic health. The purpose of genetic counseling is to help people understand how genetic conditions or mutations, such as BRCA mutations, might impact them or their families. Genetic counseling assesses risks for conditions like cancer, heart disease, or rare inherited disorders, helping individuals make informed decisions about their health and future. Counselors also explain preventive strategies, early detection measures, and treatment possibilities for those at risk. Genetic tests can determine if someone carries a harmful BRCA mutation, and results may be positive (indicating a mutation), negative (no mutation found), or uncertain (variant of uncertain significance) (National Cancer Institute, 2024). For individuals with a BRCA mutation, cancer risk can be managed through enhanced screening, risk-reducing surgery, or medication (National Cancer Institute, 2024). Treatment options for cancers with BRCA mutations may include chemotherapy agents like cisplatin or PARP inhibitors, which are effective due to the mutations causing defective DNA repair (National Cancer Institute, 2024).
EGFR
Another example of the significance of genomics is the development of drugs that target epidermal growth factor receptor (EGFR) mutations in specific types of lung cancer. EGFR-positive lung cancer accounts for 10-15% of lung cancer cases in the United States and is most commonly found in the adenocarcinoma subtype of non-small cell lung cancer (NSCLC) (American Lung Association, 2024). Most lung cancers are not hereditary, but certain types are driven by specific genetic mutations, such as those in the EGFR gene. EGFR mutations, commonly found in exons 19 and 21, lead to uncontrolled cell growth and are more prevalent in non-smokers, women, and individuals of East Asian descent (Sousa et al., 2020; Ellis, 2024). Targeted therapies, such as tyrosine kinase inhibitors (TKIs) have revolutionized treatment for EGFR-mutated lung cancer (Linardou et al., 2009). These drugs work by blocking the signaling pathways activated by EGFR mutations, significantly improving PFS and ORR compared to traditional chemotherapy. As a result, genetic testing for EGFR mutations is now a standard recommendation for patients with advanced NSCLC to determine the best personalized course of treatment (Ellis, 2024).
EGFR in the clinic
A study led by Sousa et al. (2020) highlights the critical role of advanced genomic techniques in personalized clinical treatment for lung cancer. Around 3% of NSCLC patients have rare or novel EGFR mutations that standard real-time PCR-based tests, like the cobas® EGFR Mutation Test and Idylla™ EGFR Mutation Assay, cannot detect (Sousa et al., 2020). These mutations were discovered through sequencing methods, which go beyond the limitations of targeted PCR panels designed to identify the most common EGFR mutations, such as exon 19 deletions and the L858R mutation in exon 21 (Sousa et al., 2020).
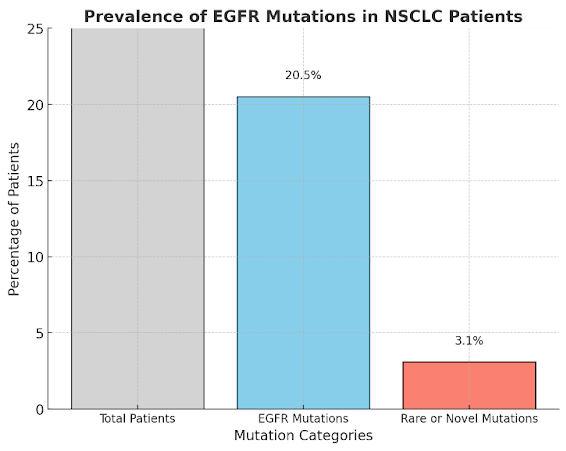
Figure 3: The prevalence of epidermal growth factor receptor (EGFR) mutations in non-small cell lung cancer (NSCLC) patients based on the study by Sousa et al. (2020). It shows the percentages of total patients, those with EGFR mutations, and those with rare or novel mutations.
By using genomic sequencing, researchers identified previously unknown single mutations and compound mutations, including complex variants and unique combinations of doublet mutations. These rare mutations carry significant implications for treatment because they can influence how patients respond to EGFR-targeted TKIs (Sousa et al., 2020). While real-time PCR assays are highly sensitive and effective at detecting frequent mutations, their focus on common variants means they might overlook rarer or atypical ones, potentially leading to less effective treatment plans. Sequencing-based methods fill this gap by providing a more comprehensive picture of a tumor’s genetic profile, giving oncologists critical insights into tumor biology. This is particularly important for patients with rare mutations, as their response to TKIs can vary depending on the specific alteration. For instance, exon 19 deletions typically predict sensitivity to TKIs, while certain rare exon 20 insertions are associated with resistance, complicating treatment options (Sousa et al., 2020). By uncovering these less common mutations, sequencing approaches ensure that treatment decisions are more precise and tailored to each patient’s unique genetic makeup (Sousa et al., 2020). Genomics enables precision medicine in cancer treatment, emphasizing the need for comprehensive sequencing to uncover actionable genetic insights that standard methodologies might overlook. By enhancing our understanding of rare genetic profiles, such research facilitates personalized and potentially more effective therapeutic strategies for lung cancer patients.
Genomics and Cardiovascular Health
Different sequencing methods have expanded understanding of genomic regions linked to congenital heart diseases, which are often connected to genetic syndromes, coronary artery disease, and other heart conditions like nonischemic cardiomyopathies and channelopathies (Novelli et al., 2010). This progress in genomic medicine is transforming our knowledge of cardiovascular disease, one of the leading causes of death across the world. Cardiovascular medicine spans a wide range of conditions, from congenital metabolic defects to complex adult-onset diseases. For those over 35-40, cardiac death is often related to ischemic cardiomyopathy, a result of atherosclerosis that can lead to heart attacks (Novelli et al., 2010). As with all areas of medicine, precise classification and genetic characterization of cardiovascular diseases are essential for advancing understanding and treatment (Novelli et al., 2010).
Genome-wide Association Studies
Genome-wide association studies (GWAS) examine genetic variants across many individuals' genomes to identify relationships between genotypes and phenotypes (National Human Genome Research Institute, 2020). Over the past decade, GWAS have revolutionized the understanding of complex diseases, uncovering numerous genetic associations with human traits and conditions. These studies have been particularly useful in identifying genetic factors for diseases such as asthma, cancer, diabetes, heart disease, and mental illnesses (National Human Genome Research Institute, 2020). Advances like the Human Genome Project (National Cancer Institute, n.d. -a) and the International HapMap Project (National Human Genome Research Institute, 2012) have enabled the scanning of multiple genomes, making such studies possible. GWAS have also led to the discovery of new disease susceptibility genes and biological pathways that are important for clinical care. Notable achievements of GWAS in medicine include identifying genetic associations for conditions like age-related macular degeneration, type 2 diabetes, and Parkinson’s disease (National Human Genome Research Institute, 2020). To conduct a GWAS, researchers compare the DNA of individuals with and without a disease, using automated machines to analyze single nucleotide polymorphisms (National Human Genome Research Institute, 2020; Tam et al., 2019). When a genetic variation is found to be significantly associated with a disease, it helps to narrow down the region of the genome responsible for the condition. The National Institutes of Health supports GWAS through collaborations and funding, resulting in large-scale research projects (National Human Genome Research Institute, 2020). Data from these studies is deposited into databases such as Database of Genotype and Phenotype (dbGaP), providing valuable resources for the broader scientific community to advance medical knowledge (National Human Genome Research Institute, 2020). While GWAS have advanced our understanding of genetic contributions to complex diseases and hold great promise for personalized medicine, ongoing research and careful interpretation of findings are essential to fully realize their potential in improving clinical care.
Tracking Infectious Diseases
Insights from genomics are used in monitoring the transmission of viruses and in modern vaccine technologies to prevent their spread. Advancements in sequencing technology (e.g., NGS) and data analytics have revolutionized microbial genome analysis. By making sequencing faster and more cost-effective, rapid public health responses can be supported. Genomic analysis is becoming the standard for identifying and controlling infectious diseases, and it enhances national surveillance by providing crucial insights into pathogen spread and antimicrobial resistance (AMR). Additionally, in cases such as outbreaks, epidemics, or even pandemics, whole genome sequencing rapidly identifies pathogen virulence factors, maps transmission pathways, and locates outbreak sources. Integrating genomic data with epidemiological information, as promoted by the One Health initiative, strengthens disease surveillance across humans, animals, and the environment, revealing how AMR evolves and spreads across species and ecosystems (Bianconi et al., 2023). Genome sequencing is also essential for investigating hospital outbreaks, as it helps identify infection sources and links between cases, informing infection control strategies (Bianconi et al., 2023). As NGS technologies evolve, they offer higher-resolution methods for pathogen analysis and classification. These tools have the ability to strengthen public health responses to virus breakouts and will aid in the future of epidemiology (Gilchrist et al, 2015).
In wake of the Covid-19 pandemic, the World Health Organization recommended that nations speed up genome sequencing and share findings in an accessible database. Hence, several forums and consortiums have begun to analyze these genomes to understand the speed and source of pathogen evolution. The majority of these disclosures are shared through groups such as the Global Initiative on Sharing all Influenza Data (GISAID), created to develop an international exchange for all clinical data breakthroughs, but is now solely used for COVID-19 information (Saravanan et al, 2022). More than 5.7 million sequences were used in 200 countries. These databases vary as they contain data from diverse sequencing methods, which could lack quality control caused by sequences shorter or longer than genome reference. All of these databases fortunately helped scientists to decipher the Sars-CoV-2 mutations and track outbreaks across the world (Saravanan et al., 2022). Genomics played a critical role during the COVID-19 pandemic, where NGS helped track viral variants, accelerate vaccine development, and monitor spread. Scientists continue to monitor changes of the evolving SARS-COV-2 virus to predict autoimmune illnesses and prevent recurrences. Rapid genome sequencing now enables faster identification and characterization of pathogens, previously demonstrated with the Zika and Ebola viruses, both examples of filoviruses, and is essential for tracing infection sources in healthcare settings (Di Paola et al., 2020). It also plays a critical role in understanding and managing filoviruses and provides valuable insight for clinicians, epidemiologists, and public health responders. Sequencing technology advancements now enable rapid, large-scale data generation to improve outbreak responses. Beyond basic viral genome sequencing, genomics explores viral transcriptomics, host immune responses in acute and persistent infections, and the characterization of animal models and natural reservoirs. Additionally, metagenomic sequencing aids in discovering novel filoviruses, while reverse genetics systems allow quick functional analysis of newly sequenced filoviruses (Di Paola et al., 2020).
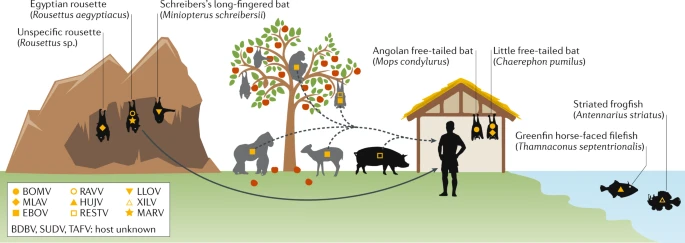
Figure 4: Example of filovirus host reservoirs and spreading from animals to humans across oceans, land, and mountainous terrain. Figure taken from Di Paola et al. (2020).
Antimicrobial Resistance
As one of the world’s greatest health and development threats, AMR is mainly responsible for 4.95 million deaths worldwide. It continues to affect low-middle income countries, makes infections harder to treat and medical procedures such as chemotherapy and surgery riskier. As estimated by the World Bank, it has cost $1 trillion in additional healthcare costs, as well as increasing the need for additional measures to ensure access to new vaccines and medicines (World Health Organization, 2023). Genomic sequencing can help to identify AMR strains of bacteria without the need for cultures (Bianconi et al., 2023).
Diagnostics
Traditionally, laboratories use culture-based methods to test diagnostic specimens. However, the time taken to grow the specimens and gather the correct information results in delays in a correct diagnosis being made. Genomic data offers faster, more accurate diagnoses, aiding in both patient care and disease surveillance. An example of this is the T2Candida Panel, which is designed to detect sepsis-causing fungal pathogens in blood. Results are provided 3 to 5 hours after the blood draw (Monday et al., 2022). This is in comparison to blood cultures which have an average time of 2-3 days for positivity and 5 days for negative results, typically determined by active or inactive growth. The speed at which results are available presents with better patient outcomes, decreasing the Candida mortality rate from 40% to 11%. (i.e., the speed of getting the results is vital for the outcome) (T2Biosystems, n.d.). Clinical microbiology has been transformed by the integration of genomics, driven by recent advances in high-cost sequencing technologies that are now cost-effective and widely accessible. This shift allows microbiologists to overcome challenges of old and prolonged methods.
The Human Microbiome
In the understanding of the human gut, shotgun metagenomics reveals taxonomic and functional insights into microbial communities, helping to analyze their roles in health and disease. However, these analyses often rely on high-quality reference databases, highlighting the need for comprehensive genome collections like the Human Microbiome Project (HMP) and the Human Gastrointestinal Bacteria Genome Collection (HGG) (Almeida et al., 2019). Advances in molecular techniques, including 16S rRNA sequencing, whole-genome sequencing, and metagenomics, have permanently shifted microbiology by discovering the incredible diversity and functions of microorganisms (Cox et al., 2013). With advanced and thorough sequencing, researchers are exploring the human microbiota (the community of microbes in the body) and discovering its critical role in immune health, involvement in infections with unknown origins, and previously unexpected connections to diseases (e.g. inflammatory bowel disease, Type 2 diabetes and Parkinson’s disease) (Manos, 2022). These tools enable in-depth study of microbiota, though challenges remain. Borrowing additional techniques from environmental microbiology may help clarify how specific microbes contribute to disease amid the complex microbial interactions within the human host (Cox et al., 2013).
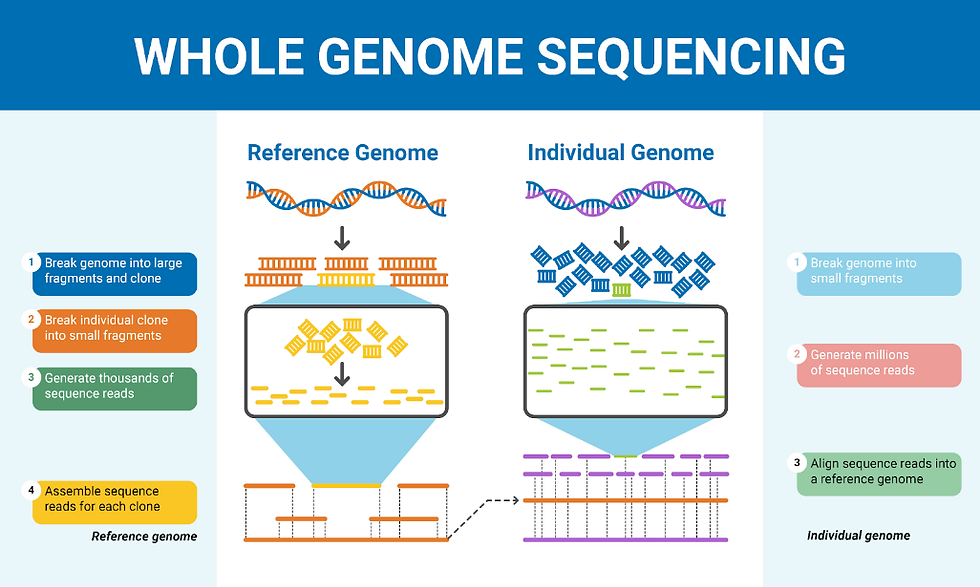
Figure 5: The difference between sequencing reference and individual genomes. Figure taken from Colby (n.d.).
Transcriptomics
Transcriptomics is the study of the transcriptome, which is the complete set of RNA molecules produced from the genome at any given time. The transcriptome is generated by the process of transcription where an RNA copy is created from a gene's DNA sequence. The idea of transcriptomics testing was introduced in 1996 by Charles Auffray (Piétu et al. 1999). The transcriptome unveils information about the way genes are working and whether proteins are being produced and expressed. The advancement of RNA-based sequencing technologies has significantly propelled the field of transcriptomics, enabling the detection and quantification of various RNA molecules including transfer RNA (tRNA), messenger RNA (mRNA), and ribosomal RNA (rRNA) (Micheel et al., 2012). Insights provided from transcriptomic analyses can be used to help clinicians decide the best course of treatment for patients, reducing costs from unnecessary treatments and increasing their chances of survival when life is at risk.
Transcriptomics Methods
Transcriptomics testing is mainly achieved by microarrays and RNA-sequencing (RNA-seq) which both produce high throughput data. RNA-seq is the most accurate and efficient of the two. During RNA-seq, the RNA is extracted, fragmented, and turned into complementary DNA (cDNA). This cDNA is then sequenced using a high-throughput platform (Sharma & Vogel, 2014). Some benefits of RNA-seq are that it covers an extremely broad range of data, it is very sensitive, it does not require probes, and it can reveal the entire transcriptome instead of just select transcripts (Sanger, n.d.). RNA-seq can also identify spliced genes and allele specific gene expression (Kukurba & Montgomery, 2015). An example of the scale of RNA-seq data is an experiment by Mortazavi et al. (2008), designed to map and quantify mouse transcriptomes, which produced 41-52 million mapped 25-base pair reads for certain mouse tissues. This shows the vastness of RNA-seq. However, drawbacks of RNA-seq include the possibility of tissue degradation, the instability of RNA, and the cost.
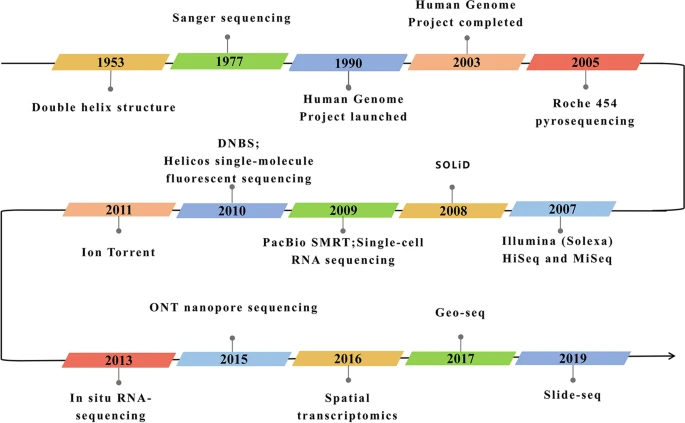
Figure 6: The development of RNA sequencing technologies in context. Figure taken from Hong et al. (2020).
Finally, microarray testing is done by binding thousands of millions of nucleic acids that are composed into a chip. That chip is then exposed to DNA or RNA which binds to the chip through complementary base pairing. This creates light that is detected by the machine, as seen in Figure 7 (Govindarajan et al., 2012). A drawback to microarray testing is that it does a poor job at detecting smaller and more specific genetic variations in comparison to RNA-seq.
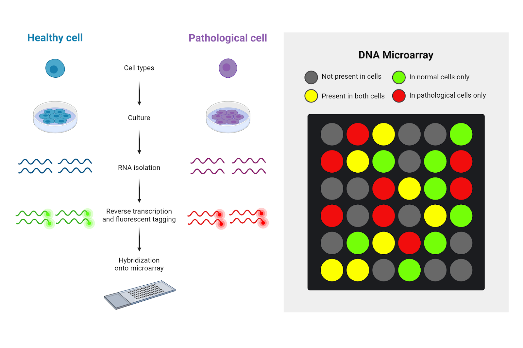
Figure 7: Process steps of a DNA microarray with context of its fluorescent light. Figure taken from Graves (2024).
Overall, a downside of transcriptomics is the lengthiness of its turnaround times. Therefore, this is not a useful technology if you know which gene(s) you are looking at. In a clinical situation where only a few genes are being analyzed, it is significantly cheaper and quicker to do PCR testing. However, if all or many genes are being analyzed, then RNA-seq or microarrays are the best technologies to use. With both of these technologies, a key analytical step is an enrichment analysis. This is an analysis to determine whether a set of genes is over or underrepresented in a set of data (Mazzoccoli et al., 2013). It does this by considering a list of all genes and the common number of those genes.
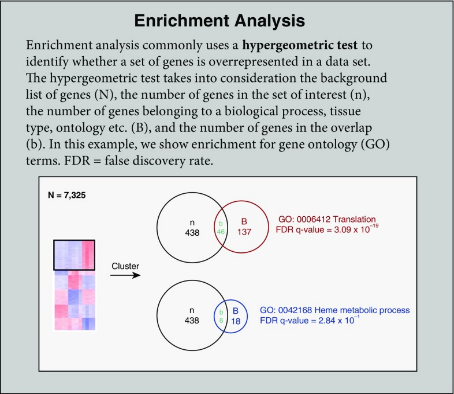
Figure 8: Description of the enrichment analysis process. Figure taken from Koch et al. (2018).
Oncotype Dx
The Oncotype Dx is a 21-gene predictive and diagnostic assay which was created for early-stage breast cancer patients that estimates what the probability of cancer returning (Suplitt et al., 2021). After the test is run, scores can range anywhere from 0-100, with 0 meaning the cancer will not return and 100 being that the cancer will return. A low score is considered anywhere from 0-17 (Schaafsma et al., 2021). Patients who receive a low score will usually decide not to continue doing chemotherapy or some other form of treatment. Economically, this can be efficient because patients have the opportunity to save money on treatments. Not only that, but the wellbeing of patients will be preserved as chemotherapy is a grueling treatment that takes a toll on patients. A high score would be considered anywhere from 30 and upwards (Schaafsma et al., 2021). However, this is dependent on the judgment of different clinicians. Considering a score of 30 high enough to continue treatment ensures the patient’s safety. Gathering results of a high score lets the patient know they should continue treatment and could increase their chance of survival. However, there are some intermediate scores that have some uncertainty in terms of the best treatment options (Schaafsma et al., 2021).
Inflammatix
The Inflammatix tests hard to diagnose patients who have an infection to determine the specifics of their infection. Uncertainties like whether the illness is viral or bacterial or a non-infectious disease can be determined within 30 minutes (Kostaki et al., 2022). These timely results help to identify a patient’s condition as efficiently as possible. Inflammatix first tests a patient's blood to determine whether the illness is non-infectious, bacterial, or viral. Then, from the blood sample, a gene expression profile is read. From this gene expression profile, the level of infection is determined. Inflammatix’s TriVerity test is not yet FDA approved but, ultimately, is a huge step forward in big data considering its combination of gene expression data with machine learning algorithms to efficiently determine a patient's condition (Inflammatix, n.d.; Kostaki et al., 2022).
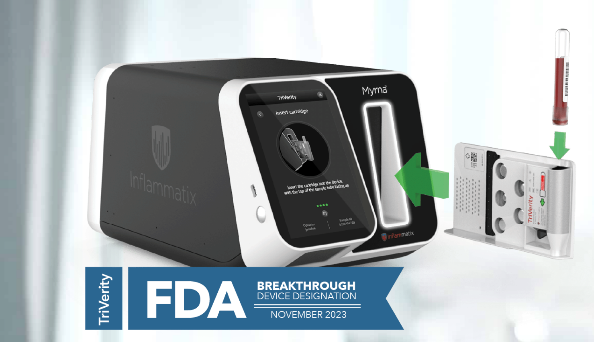
Figure 9: An Inflammatix device. Figure taken from Inflammatix (n.d.).
Transcriptomics in Context
These various transcriptomics testing methods help to gain more insight surrounding numerous different diseases. For example, Hunter disease is a rare disease, usually found in males, where patients could potentially have growth problems and heart issues (Mazzoccoli et al., 2013). Transcriptomics compares the transcriptome of healthy patients to patients with the disease to determine the genes and pathways that are being altered. By doing this, clinicians can determine what treatment patients might need in the future. Usually, in the case of Hunter's Disease that would be enzyme replacement therapy (Concolino et al., 2018). The same can be seen with McArdle disease. Patients who have McArdle disease have a genetic disorder that affects the skeletal muscles of a patient (Khattak et al., 2023). McArdle disease is usually undiagnosed because of its overlapping symptoms with other conditions. Transcriptomics technologies can identify specific biomarkers or patterns in patients which will be able to tell doctors whether the patient has McArdle disease and needs medication or therapy. Transcriptomics and all of its diverse testing methods aid patients and clinicians by detecting diseases early, understanding the nature of diseases, and developing disease treatments.
Proteomics
The proteome encompasses the entire set of proteins expressed by a cell, tissue, or organism. It is inherently complex due to the potential for proteins to undergo various post-translational modifications, such as glycosylation, phosphorylation, acetylation, and ubiquitylation. Proteins can also have different spatial configurations, intracellular localizations, and interactions with other proteins and molecules. This complexity poses challenges for developing proteomics-based tests. The proteome can be analyzed using mass spectrometry and protein microarrays (Ahrens et al., 2010; Wolf-Yadlin et al., 2009).
Mass Spectrometry-based Proteomics
Mass spectrometry-based proteomics has made significant advances in recent years, enhancing sensitivity and robustness. This progress makes it feasible to integrate these systems into clinical environments. These instruments, which include liquid chromatography front-ended and MALDI imaging-based systems, surpass the capabilities of simpler mass spectrometers used in clinical labs for drug monitoring. Unlike antibody-dependent detection methods, direct protein mass spectrometry detects analytes without the requirement for antibodies, providing an unbiased approach. Recent developments allow for mass spectrometric analysis of smaller sample amounts (including single cells or organelles), offering opportunities to understand biochemical diversity within cancers (Roehrl et al., 2021). Proteome-based diagnostics provide insights into cell signaling activation by measuring total and post-translationally modified proteomes, which cannot be accessed through genomic or transcriptomic analyses.
Proteomics Experiments
One can gain key insights into the composition, regulation and function of molecular pathways using mass spectrometry (Cravatt, et al., 2007). However, proteomic experiments generate vast amounts of data, and for these data sets to be meaningful or to inspire hypotheses, carefully designed experimental systems and controls are crucial. Proper use of controls enables researchers to narrow down proteomic observations to a manageable number of proteins that exhibit changes in abundance, activity, or post-translational modification in the studied model. When executed correctly, mass-spectrometry-based proteomics should reveal a set of proteins linked to a specific cellular or physiological process. However, understanding the function of these proteins requires targeted follow-up studies using complementary experimental approaches. For example, RNA interference (RNAi) can be used to disrupt the production of any protein in cells and organisms. RNAi is compatible with large-scale screening, allowing researchers to quickly validate the biological function of hundreds to thousands of candidate proteins. The best way to illustrate the synergistic relationship between mass-spectrometry-based proteomics and RNAi is to view the former as a hypothesis-generating tool and the latter as a method for testing these hypotheses. Together, these methods connect proteomic observations with a function or phenotype.
Proteomics in Medicine
Consider two identical twins: their genomes are the same, but their unique fingerprints distinguish them (Roehrl et al., 2021). Similarly, imagine two cancer cells with identical genomic alterations. Although sequencing might find them identical, their biochemical signaling states could be vastly different, affecting their response to targeted drugs. A patient's cancer biopsy could undergo both genomic and proteomic profiling, classifying cancers and metastases into subtypes, assessing signaling pathways, and identifying neoproteins/neoepitopes to target with treatments. Integrated proteogenomic analysis can predict the most sensitive molecular targets and identify resistance mechanisms, aiding treatment selection.
Proteome-based diagnostics also hold promise for diseases with poorly understood etiologies, such as autoimmune diseases. Proteomics can differentiate between patients within a disease class, cataloguing autoantigens and antibody signatures in a way that cannot be achieved with traditional diagnostics. Focusing on proteomics will impact diagnostics, risk prediction, disease monitoring, and therapy selection. This requires changes in clinical practice and education about proteomics' capabilities and limitations (Roehrl, et al., 2021).
The Cancer Genome Atlas, coordinated by the US National Cancer Institute, has enhanced our genomic understanding of many cancers. The Clinical Proteomic Tumor Analysis Consortium (CPTAC) aims to catalogue proteomic changes across various cancer types and develop technologies for deep proteomic profiling (National Cancer Institute, n.d. -b). Additionally, the Human Proteome Organization (HUPO) recently launched the Pathology Pillar of the Human Proteome Project (Human Proteome Organization, n.d.). These projects show an increased interest in proteomics as the technologies evolve.
Metabolomics
Metabolomics is the identification and quantification of all small molecule metabolites that exist within cells, tissues, and organisms. Metabolomics has a wide range of applications in fields such as medicine, environmental science, and food quality (Courant et al., 2014). In medicine, metabolomics is an incredibly important tool for understanding the mechanisms of drugs and the causes of diseases (Courant et al., 2014). Since each disease has a specific metabolic profile, metabolomics is also used to investigate biomarkers of diseases to improve diagnosis (Jacob et al., 2017).
As of now, there are many limitations to clinical efficacy for much of the population, such as misdiagnosis and negative side-effects from medication (Jacob et al., 2017). Metabolomics can be used to identify biomarkers, which are molecules found in cells, tissues, blood, and other bodily fluids that signify whether a certain condition or disease exists in the sample (National Cancer Institute, n.d. -c). Biomarker identification would aid in the development of personalized treatment plans, especially for conditions that have differing symptoms due to being a metabolic or multifaceted disease such as diabetes, cardiovascular disease, and chronic kidney disease (Jacob et al., 2017; Shi et al., 2023).
Metabolomics Methods
Historically, there have been three main methods of metabolomics analysis through mass spectrometry. The first method was metabolite targeted analysis. The second was metabolic profiling, and the third was metabolic fingerprinting (Shulaev, 2006). However, due to advancements in technologies, both metabolic profiling and fingerprinting have become the same approach.
Targeted analysis is hypothesis-driven and identifies known metabolites (Courant et al., 2014). It deals with a single or specific set of compounds, so it can be analyzed quantitatively or semi-quantitatively (Roberts et al., 2012). Metabolic fingerprinting, or untargeted metabolomics, is not hypothesis-driven and instead tracks patterns and compares changes in metabolomes (Courant et al., 2014; Cao et al., 2022). As untargeted metabolomics is a comprehensive analysis of the entire metabolome, including unknowns, it is analyzed qualitatively and allows for new metabolic discoveries (Roberts et al., 2012).
The techniques most often used to analyze metabolite profiles are nuclear magnetic resonance (NMR), gas chromatography-mass spectrometry (GC-MS), liquid chromatography (LC-MS), and capillary electrophoresis-mass spectrometry (CE-MS) (Shulaev, 2006). NMR is useful for rapid analysis and high-resolution results; however, it has low sensitivity and complicated spectra (Shulaev, 2006). GC-MS is very sensitive and has a large linear range; however it is slow, and the samples could be too volatile to analyze with this technology (Shulaev, 2006). LC-MS can have a large sample, but it is slow to analyze the metabolites. CE-MS has rapid analysis and needs small samples, but it is difficult to reproduce its retention time (Shulaev, 2006).

Figure 10: Typical workflow of mass spectrometry-based metabolomics. The first (1) step for metabolomics is to prepare and extract the sample. The second (2) is to separate the metabolites with gas or liquid chromatography. The third (3) step is to ionize the metabolites with the use of an ion source. The fourth (4) is to separate the ions based on their mass-to-charge (m/z) ratio with a mass spectrometer. The final (5) step is to identify the metabolites. They can be identified by both their retention time (RT) and mass-spectrometry (MS) signature. Figure taken from Alseekh et al. (2021).
Temprosa et al. (2021) introduced an online tool called Consortium of Metabolomics Studies (COMETS) Analytics that allows for convenient and fast analysis of metabolomics data. It provides standard analyses for all cohorts and performs accurately, and, additionally, it doesn’t need specific software to operate (Temporosa et al., 2021). It has also allowed researchers to compile a “metabolite dictionary that links metabolite names across 60 different data sets” (Temprosa et al., 2021).
It is difficult to identify most molecules due to a lack of reference spectra. Van der Hooft et al. (2016) developed MS2LDA, a tool to aid in metabolomic fingerprinting. It was able to identify patterns and losses to map out molecular relationships and build a reference spectra (van der Hooft et al., 2016). This tool would be useful in identifying compounds in untargeted metabolomics.
Intelligent Knife
The intelligent knife (iKnife) is a mix of an electrosurgical knife, which uses electrical currents, and rapid evaporative ionization mass spectrometry (REIMS) to precisely and accurately identify molecules and tissues. The use of the electrosurgical knife on tissue allows for the release of surgical aerosol. Then, mass spectrometry is used to immediately analyze the vaporized tissue to diagnose intraoperatively (Jacob et al., 2017).
Certain cancers can be surgically removed by identifying the tumors and cutting them out. However, pre-surgical analysis and diagnosis of cancerous tissue and normal tissue is extremely complicated, tedious, and laborious (St John et al., 2017). The iKnife is a crucial asset in improving the accuracy and efficiency of cancer operations leading to higher chances of survival for the individual affected (Phelps et al., 2018).
Tzafetas et al. (2020) investigated the ability of the iKnife to assist with the surgical identification and removal of tumors causing cervical disease. This technology can detect the cancerous tissue due to the “dysregulation of lipid metabolism” that can be related with cancers (Tzafetas et al., 2020). The researchers found the iKnife to be extremely specific and accurate in differentiating types of tissues and determining whether the tissue was cancerous; they suggest the iKnife is useful for diagnosis during surgical operation.
Phelps et al. (2018) examined how effectively REIMS can analyze gynecological tissues during surgery and distinguish between cancerous tissue and normal tissue. The researchers used 335 tissue samples to test REIMS in its identification. They found it to have a 97.4% sensitivity and 100% specificity in distinguishing between ovarian cancer and normal tissue (Phelps et al., 2018). It was also found to have a 90.5% sensitivity and 89.7% specificity in differentiating borderline ovarian tumors (BOT) from ovarian cancer (Phelps et al., 2018). REIMS could even identify five lipid species to be more abundant in ovarian cancer tissue compared to normal tissue or BOT (Phelps et al., 2018). This technology was able to successfully analyze metabolomics and lipidomics data to efficiently diagnose gynecological tissue intra-operatively.
Drug Development
Mukherjee et al. (2016) evaluated how the metabolomics of medical plants and herbal products, specifically in Ayurveda, can aid in the development of personalized medicine. Ayurvedic medicine is an ancient practice in India that specializes in personalized healthcare and treatment. Metabolomic profiling “not only identifies the metabolites relative to distribution of compounds with each other but also compares the nature of compounds” (Mukherjee et al., 2016). This method can be used to identify the biological activities of metabolites in these herbs and understand their therapeutic potential (Mukherjee et al., 2016). The identification of the biochemical properties of the plants could be a powerful tool for drug discovery from natural resources.
Discussion
Genomics has revolutionized cancer research by identifying DNA mutations that drive tumor growth, aiding advances in precision medicine. Mutations in BRCA1 and BRCA2 genes increase the risk for breast and ovarian cancers, but they also make tumors susceptible to treatments like PARP inhibitors. Clinical trials have shown that targeting these mutations can lead to higher response rates and progression free survival in patients with BRCA-mutated cancers. Beyond cancer, genomics plays a huge role in GWAS, which identify genetic factors underlying complex diseases and in diagnosing and tracing infectious diseases. Complementing genomics, transcriptomics reveals information regarding how much each gene is being expressed and transcriptome-based technologies such as the Oncotype Dx are able to inform treatment decisions in the oncology sphere. While transcriptomics focuses on analyzing RNA to understand its impact on health, metabolomics examines small molecules and leans towards phenotypic analysis. Technologies such as the iKnife use metabolomics to identify cancerous tissues during surgery, leading to better outcomes for patients. In addition, proteomics is transforming medicine by enhancing cancer diagnostics through protein-based insights into cell signaling, cancer classification, and drug response prediction. Together, these types of omics information pave the way for personalized medicine, where treatments are tailored to the genetic and molecular profiles of individual patients. With a clearer understanding of disease pathways at the molecular level, researchers can develop new drugs that specifically target those pathways, reducing side effects and improving treatment effectiveness.
Limitations
While omics technologies hold great promise for advancing our understanding of biological systems, the challenges posed by large data sets cannot be overlooked. Though omics technologies generate vast amounts of data, the complexity of these findings leads to challenges in interpretation (Choi & Pavelka, 2012). Biological systems are complex and interconnected, making it difficult to translate raw data into clear insights. Even though there is a lot of data, extracting meaningful information requires expertise in a variety of fields, a skill not available to all. The abundance of molecular information can saturate potential users, presenting too many targets or pathways to explore. As a result, there is a gap between the data we generate and the knowledge we can gain and use in understanding biology. Advanced AI models can capture intricate patterns in large data sets. However, their complexity often leads to a lack of interpretability, making it challenging for researchers to understand how specific predictions are made. This "black box" nature of AI models limits their usefulness in providing actionable biological insights and hinders their acceptance in the scientific community (Savage, 2022).
Privacy
In the world of big data, mass collection of information helps scientists better their understanding of health and disease which helps clinicians to improve patient care. However, there is potential for privacy breaches both for individual information and that of an organization. Sometimes, to get patients to participate and share their information, organizations will offer an incentive to participate. Frequent incentivizing strategies that organizations use are offering money, offering a way to save money, providing healthcare benefits, handing out gift cards or vouchers, and providing patient access to results of the study being conducted. These benefits are sometimes not enough for participants to risk having their personal information exposed. Ensuring that data is kept private becomes increasingly difficult as data is shared around the world. There have been situations where clinicians have assured patients that their information was anonymous, only to find out from computer scientists that their information could be traced back and used to identify specific individuals (Tene & Polonetsky, 2012).
Storage
With the growth of big data and its prevalence in modern medicine, there are concerns regarding storage for the sheer amount of data being produced. Traditional databases are not able to handle the “complex data sources, diverse structures, huge scale, rapid growth, and multimodality” of big data (Chen et al., 2023). The implementation of cloud-based platforms has benefits of increased storage space and efficient data management; however, it poses issues of privacy and is limited to the network bandwidth in terms of speed (Awrahman, 2022). Some projects utilizing big data need much more storage than others, such as the Human Connectome Project, which collects scans and images of the brain. This presented huge data management challenges, which resulted in an amalgamation of multiple datasets and data-processing approaches to handle the amount of data (The DataLad Handbook, 2023). Moving forward, it is crucial to find a viable, concrete solution for storage as the world of big data continues to expand.
Expense and Accessibility
Even as big data has helped enable personalized medicine by identifying the best and most efficient methods of treatment for patients with specific issues, it has also been expensive to work with due to the complex nature of data collection and analysis. It requires investments in storage solutions, analytics tools, cybersecurity, and staff expertise (Partida, 2023). There are also continual expenditures for storing and processing data, a challenge for non-profit organizations and the education sector (Harvard Online, 2024). Expensive omics technology leaves those in low-income countries at a particular disadvantage. Those in low-income countries with poor medical care are often the ones who would most benefit from these improvements, but the financial costs leave these advancements inaccessible.
Future Directions
Although omics technologies work effectively separately, when brought together they can yield even better results. Multi-omics is the merging of multiple types of omics technologies and is becoming increasingly common in medical research. As omics technologies, both individually and together, continue to expand, they allow for the continued growth of individualized treatment and medication. With many treatments having negative side effects or poor efficacy, precision medicine is incredibly important for getting the right drugs to the right patients. For conditions such as cancer, this type of personalized treatment is essential and lifesaving.
Conclusion - Omics Technologies
The rise of big data in medicine has had a huge impact on the analysis of genetic information and the development of personalized medicine. Although the four discussed omics technologies vary in applications, together they provide a comprehensive understanding of biological systems. Genomics play a crucial role in understanding the genetic foundation of diseases by analyzing the genome to identify mutations. This knowledge allows for more accurate diagnoses, personalized treatments, and the potential to discover new therapeutic targets. Applications of genomics include defining cancer types based on their genetic profile, genetic counseling, and addressing rare conditions. Transcriptomics technologies are used to understand the sum of an organism’s RNA transcripts. Using this technology, researchers gain further understanding of disease by comparing specific pairs of samples (e.g., healthy vs diseased) and looking at the differences in gene activity between the two. Metabolomics aids in the accuracy of diagnosis and treatment through the identification of significant biomarkers of diseases. The iKnife has revolutionized cancer operations and increased the patients’ chances for survival by using REIMS to distinguish between tumors and non-cancerous tissue intra-operatively. Proteomics, the large-scale study of the structure, function, and interactions of proteins, also helps to identify biomarkers for diseases and provides insights into the molecular interactions of health and disease. As these technologies evolve, they will play a key role in personalized medicine, paving the way for more accurate and effective treatments.
References
Ahrens, C. H., Brunner, E., Qeli, E., Basler, K., & Aebersold, R. (2010). Generating and Navigating Proteome Maps Using Mass Spectrometry. Nature Reviews. Molecular Cell Biology, 11(11), 789–801. https://doi.org/10.1038/nrm2973
Almeida, A., Mitchell, A. L., Boland, M., Forster, S. C., Gloor, G. B., Tarkowska, A., Lawley, T. D., & Finn, R. D. (2019). A New Genomic Blueprint of the Human Gut Microbiota. Nature, 568(7753), 499–504. https://doi.org/10.1038/s41586-019-0965-1
Alseekh, S., Aharoni, A., Brotman, Y. et al., (2021). Mass Spectrometry-Based Metabolomics: a Guide for Annotation, Quantification and Best Reporting Practices. Nature Methods, 18, 747–756. https://doi.org/10.1038/s41592-021-01197-1
American Lung Association. (2024, September 26). EGFR and Lung Cancer. https://www.lung.org/lung-health-diseases/lung-disease-lookup/lung-cancer/symptoms-diagnosis/biomarker-testing/egfr
Audeh, M. W., Carmichael, J., Penson, R. T., Friedlander, M., Powell, B., Bell-McGuinn, K. M., Scott, C., Weitzel, J. N., Oaknin, A., Loman, N., Lu, K., Schmutzler, R. K., Matulonis, U., Wickens, M., & Tutt, A. (2010). Oral Poly(ADP-Ribose) Polymerase Inhibitor Olaparib in Patients with BRCA1 or BRCA2 Mutations and Recurrent Ovarian Cancer: a Proof-of-Concept Trial. Lancet, 376(9737), 245–251. https://doi.org/10.1016/S0140-6736(10)60893-8
Awrahman, B. J., Aziz Fatah, C., & Hamaamin, M. Y. (2022). A Review of the Role and Challenges of Big Data in Healthcare Informatics and Analytics. Computational Intelligence and Neuroscience, 2022, 5317760. https://doi.org/10.1155/2022/5317760
Bianconi, I., Aschbacher, R., & Pagani, E. (2023). Current Uses and Future Perspectives of Genomic Technologies in Clinical Microbiology. Antibiotics, 12(11), 1580. https://doi.org/10.3390/antibiotics12111580
Cao, J., Wang, Y., Zhang, Y. and Qian, K. (2022). Emerging Applications of Mass Spectrometry-Based Metabolic Fingerprinting in Clinics. Advanced Intelligent Systems., 4: 2100191. https://doi.org/10.1002/aisy.202100191
Chen, H., Song, Z., Yang, F., (2023). [Retracted] Storage Method for Medical and Health Big Data Based on Distributed Sensor Network, Journal of Sensors. https://doi.org/10.1155/2023/8506485
Choi, H., & Pavelka, N. (2012). When One and One Gives More Than Two: Challenges and Opportunities of Integrative Omics. Frontiers in Genetics, 2, 105. https://doi.org/10.3389/fgene.2011.00105
Colby, B. (n.d.) Whole Genome Sequencing. https://sequencing.com/education-center/whole-genome-sequencing
Concolino, D., Deodato, F., & Parini, R. (2018). Enzyme Replacement Therapy: Efficacy and Limitations. Italian Journal of Pediatrics, 44(Suppl 2), 120.
Courant, F., Antignac, J. P., Dervilly-Pinel, G., & Le Bizec, B. (2014). Basics of Mass Spectrometry Based Metabolomics. Proteomics, 14(21-22), 2369–2388. https://doi.org/10.1002/pmic.201400255
Cox, M. J., Cookson, W. O. C. M., & Moffatt, M. F. (2013). Sequencing the Human Microbiome in Health and Disease. Human Molecular Genetics, 22(R1), R88–R94. https://doi.org/10.1093/hmg/ddt398
Cravatt, B. F., Simon, G. M., & Yates, J. R., 3rd (2007). The Biological Impact of Mass-Spectrometry-Based Proteomics. Nature, 450(7172), 991–1000. https://doi.org/10.1038/nature06525
Dahlstrom, E. (2024, April 3). What are PARP Inhibitors? MD Anderson Cancer Center. https://www.mdanderson.org/cancerwise/what-are-parp-inhibitors.h00-159696756.html
Dai, X., & Shen, L. (2022). Advances and Trends in Omics Technology Development. Frontiers in Medicine, 9, 911861. https://doi.org/10.3389/fmed.2022.911861
Di Paola, N., Sanchez-Lockhart, M., Zeng, X., Kuhn, J. H., & Palacios, G. (2020). Viral Genomics in Ebola Virus Research. Nature reviews. Microbiology, 18(7), 365–378. https://doi.org/10.1038/s41579-020-0354-7
Ellis, R. R. (2024, October 29). EGFR Mutations in NSCLC: What Does It Mean? WebMD. https://www.webmd.com/lung-cancer/egfr-mutations-defined-nsclc
Gilchrist, C. A., Turner, S. D., Riley, M. F., Petri, W. A., Jr, & Hewlett, E. L. (2015). Whole-Genome Sequencing in Outbreak Analysis. Clinical Microbiology Reviews, 28(3), 541–563. https://doi.org/10.1128/CMR.00075-13
Govindarajan, R., Duraiyan, J., Kaliyappan, K., & Palanisamy, M. (2012). Microarray and Its Applications. Journal of Pharmacy & Bioallied Sciences, 4(Suppl 2), S310–S312. https://doi.org/10.4103/0975-7406.100283
Graves, B. (2024, February 22). Common Applications of DNA Microarrays. AAT Bioquest. https://www.aatbio.com/resources/application-notes/common-applications-of-dna-microarrays
Harvard Online. (2024, February 28). Pros and Cons of Big Data. https://www.harvardonline.harvard.edu/blog/pros-cons-big-data
History Associates Incorporated. (2021, February 10). Case Study: Leroy Hood. https://lemelson.mit.edu/sites/default/files/2021-02/LMIT_Hood_CaseStudy.pdf
Hong, M., Tao, S., Zhang, L., Diao, L. T., Huang, X., Huang, S., Xie, S. J., Xiao, Z. D., & Zhang, H. (2020). RNA Sequencing: New Technologies and Applications In Cancer Research. Journal Of Hematology & Oncology, 13(1), 166. https://doi.org/10.1186/s13045-020-01005-x
Human Proteome Organization. (n.d.). Pathology Pillar. https://www.hupo.org/Pathology-Pillar
Hutchinson L. (2010). Targeted Therapies: PARP Inhibitor Olaparib is Safe and Effective in Patients with BRCA1 And BRCA2 Mutations. Nature Reviews. Clinical Oncology, 7(10), 549. https://doi.org/10.1038/nrclinonc.2010.143
Inflammatix. (n.d.). TriVerity: Acute Infection and Sepsis Test. https://inflammatix.com/
Jacob, M., Lopata, A. L., Dasouki, M., & Abdel Rahman, A. M. (2017). Metabolomics Toward Personalized Medicine. Mass Spectrometry Reviews, 38(3), 221–238. https://doi.org/10.1002/mas.21548
Khattak, Z. E., Lam, J. R., & Ashraf, M. (2023). McArdle Disease. In StatPearls. StatPearls Publishing. https://pubmed.ncbi.nlm.nih.gov/32809620/
Koch, C. M., Chiu, S. F., Akbarpour, M., Bharat, A., Ridge, K. M., Bartom, E. T., & Winter, D. R. (2018). A Beginner's Guide to Analysis of RNA Sequencing Data. American Journal Of Respiratory Cell And Molecular Biology, 59(2), 145–157. https://doi.org/10.1165/rcmb.2017-0430TR
Kostaki, A., Wacker, J. W., Safarika, A., Solomonidi, N., Katsaros, K., Giannikopoulos, G., Koutelidakis, I. M., Hogan, C. A., Uhle, F., Liesenfeld, O., Sweeney, T. E., & Giamarellos-Bourboulis, E. J. (2022). A 29-Mrna Host Response Whole-Blood Signature Improves Prediction of 28-Day Mortality and 7-Day Intensive Care Unit Care in Adults Presenting to the Emergency Department with Suspected Acute Infection and/or Sepsis. Shock, 58(3), 224–230. https://doi.org/10.1097/SHK.0000000000001970
Kukurba, K. R., & Montgomery, S. B. (2015). RNA Sequencing and Analysis. Cold Spring Harbor Protocols, 2015(11), 951–969. https://doi.org/10.1101/pdb.top084970
Linardou, H., Dahabreh, I. J., Bafaloukos, D., Kosmidis, P., & Murray, S. (2009). Somatic EGFR Mutations and Efficacy of Tyrosine Kinase Inhibitors in NSCLC. Nature Reviews. Clinical Oncology, 6(6), 352–366. https://doi.org/10.1038/nrclinonc.2009.62
Manos J. (2022). The Human Microbiome in Disease and Pathology. APMIS: Acta Pathologica, Microbiologica, et Immunologica Scandinavica, 130(12), 690–705. https://doi.org/10.1111/apm.13225
Mazzoccoli, G., Tomanin, R., Mazza, T., D'Avanzo, F., Salvalaio, M., Rigon, L., Zanetti, A., Pazienza, V., Francavilla, M., Giuliani, F., Vinciguerra, M., & Scarpa, M. (2013). Circadian Transcriptome Analysis in Human Fibroblasts From Hunter Syndrome And Impact Of Iduronate-2-Sulfatase Treatment. BMC Medical Genomics, 6, 37. https://doi.org/10.1186/1755-8794-6-37
Micheel, C. M., Nass, S. J., Omenn, G. S. (Eds). (2012). Evolution of Translational Omics: Lessons Learned and the Path Forward. National Academies Press (US). https://www.ncbi.nlm.nih.gov/books/NBK202165/
Monday, L. M., Parraga Acosta, T., & Alangaden, G. (2021). T2Candida for the Diagnosis and Management of Invasive Candida Infections. Journal of Fungi, 7(3), 178. https://doi.org/10.3390/jof7030178
Mortazavi, A., Williams, B. A., McCue, K., Schaeffer, L., & Wold, B. (2008). Mapping and Quantifying Mammalian Transcriptomes by RNA-Seq. Nature Methods, 5(7), 621–628. https://doi.org/10.1038/nmeth.1226
Mukherjee, P. K., Harwansh, R. K., Bahadur, S., Biswas, S., Kuchibhatla, L. N., Tetali, S. D., & Raghavendra, A. S. (2016). Metabolomics of Medicinal Plants – A Versatile Tool for Standardization of Herbal Products and Quality Evaluation of Ayurvedic Formulations. Current Science, 111(10), 1624–1630. https://doi.org/10.18520/cs/v111/i10/1624-1630
National Cancer Institute. (2024, July 19). BRCA Gene Changes: Cancer Risk and Genetic Testing. https://www.cancer.gov/about-cancer/causes-prevention/genetics/brca-fact-sheet
National Cancer Institute (n.d. -a). The Cancer Genome Atlas Program (TCGA). https://www.cancer.gov/ccg/research/genome-sequencing/tcga
National Cancer Institute. (n.d. -b). Clinical Proteomic Tumor Analysis Consortium (CPTAC). https://gdc.cancer.gov/about-gdc/contributed-genomic-data-cancer-research/clinical-proteomic-tumor-analysis-consortium-cptac
National Cancer Institute. (n.d. -c). NCI Dictionary of Cancer Terms. https://www.cancer.gov/publications/dictionaries/cancer-terms/def/biomarker
National Human Genome Research Institute. (2012, May 1). International HapMap Project. https://www.genome.gov/10001688/international-hapmap-project
National Human Genome Research Institute. (2020, August 17). Genome-wide Association Studies fact sheet. https://www.genome.gov/about-genomics/fact-sheets/Genome-Wide-Association-Studies-Fact-Sheet
Neo Genomics. (n.d.). Molecular. https://neogenomics.com/pharma-services/lab-services/molecular
Ng, S. B., Buckingham, K. J., Lee, C., Bigham, A. W., Tabor, H. K., Dent, K. M., Huff, C. D., Shannon, P. T., Jabs, E. W., Nickerson, D. A., Shendure, J., & Bamshad, M. J. (2010). Exome Sequencing Identifies the Cause of a Mendelian Disorder. Nature Genetics, 42(1), 30–35. https://doi.org/10.1038/ng.499
Novelli, G., Predazzi, I. M., Mango, R., Romeo, F., & Mehta, J. L. (2010). Role Of Genomics in Cardiovascular Medicine. World Journal of Cardiology, 2(12), 428–436. https://doi.org/10.4330/wjc.v2.i12.428
Oliver, S. G., van der Aart, Q. J., Agostoni-Carbone, M. L., Aigle, M., Alberghina, L., Alexandraki, D., Antoine, G., Anwar, R., Ballesta, J. P., & Benit, P. (1992). The Complete DNA Sequence of Yeast Chromosome III. Nature, 357(6373), 38–46. https://doi.org/10.1038/357038a0
Partida, D. (2023, October 4) Top 7 Challenges of Big Data and Solutions. Datamation. https://www.datamation.com/big-data/big-data-challenges/
Phelps, D. L., Balog, J., Gildea, L. F., Bodai, Z., Savage, A., El-Bahrawy, M. A., Speller, A. V., Rosini, F., Kudo, H., McKenzie, J. S., Brown, R., Takáts, Z., & Ghaem-Maghami, S. (2018). The Surgical Intelligent Knife Distinguishes Normal, Borderline and Malignant Gynaecological Tissues Using Rapid Evaporative Ionisation Mass Spectrometry (REIMS). British Journal of Cancer, 118(10), 1349–1358. https://doi.org/10.1038/s41416-018-0048-3
Piétu, G., Mariage-Samson, R., Fayein, N. A., Matingou, C., Eveno, E., Houlgatte, R., Decraene, C., Vandenbrouck, Y., Tahi, F., Devignes, M. D., Wirkner, U., Ansorge, W., Cox, D., Nagase, T., Nomura, N., & Auffray, C. (1999). The Genexpress IMAGE Knowledge Base of the Human Brain Transcriptome: a Prototype Integrated Resource for Functional and Computational Genomics. Genome Research, 9(2), 195–209.
Roach, J. C., Glusman, G., Smit, A. F., Huff, C. D., Hubley, R., Shannon, P. T., Rowen, L., Pant, K. P., Goodman, N., Bamshad, M., Shendure, J., Drmanac, R., Jorde, L. B., Hood, L., & Galas, D. J. (2010). Analysis of Genetic Inheritance in a Family Quartet by Whole-Genome Sequencing. Science, 328(5978), 636–639. https://doi.org/10.1126/science.1186802
Roberts, L. D., Souza, A. L., Gerszten, R. E., & Clish, C. B. (2012). Targeted Metabolomics. Current Protocols in Molecular Biology, Chapter 30, Unit30.2–30.2.24. https://doi.org/10.1002/0471142727.mb3002s98
Roehrl, M. H., Roehrl, V. B., & Wang, J. Y. (2021). Proteome-Based Pathology: the Next Frontier in Precision Medicine. Expert Review of Precision Medicine and Drug Development, 6(1), 1–4. https://doi.org/10.1080/23808993.2021.1854611
Rose, M., Burgess, J. T., O'Byrne, K., Richard, D. J., & Bolderson, E. (2020). PARP Inhibitors: Clinical Relevance, Mechanisms of Action and Tumor Resistance. Frontiers in Cell and Developmental Biology, 8, 564601. https://doi.org/10.3389/fcell.2020.564601
Saravanan, K. A., Panigrahi, M., Kumar, H., Rajawat, D., Nayak, S. S., Bhushan, B., & Dutt, T. (2022). Role of Genomics in Combating COVID-19 Pandemic. Gene, 823, 146387. https://doi.org/10.1016/j.gene.2022.146387
Savage N. (2022). Breaking into the Black Box of Artificial Intelligence. Nature, 10.1038/d41586-022-00858-1. https://doi.org/10.1038/d41586-022-00858-1
Schaafsma, E., Zhang, B., Schaafsma, M., Tong, C. Y., Zhang, L., & Cheng, C. (2021). Impact of Oncotype DX Testing On ER+ Breast Cancer Treatment and Survival in the First Decade of Use. Breast Cancer Research, 23(1), 74. https://doi.org/10.1186/s13058-021-01453-4
Sharma, C. M., & Vogel, J. (2014). Differential RNA-Seq: the Approach Behind and the Biological Insight Gained. Current Opinion in Microbiology, 19, 97–105. https://doi.org/10.1016/j.mib.2014.06.010
Shi, C., de Wit, S., Učambarlić, E., Markousis-Mavrogenis, G., Screever, E. M., Meijers, W. C., de Boer, R. A., & Aboumsallem, J. P. (2023). Multifactorial Diseases of the Heart, Kidneys, Lungs, and Liver and Incident Cancer: Epidemiology and Shared Mechanisms. Cancers, 15(3), 729. https://doi.org/10.3390/cancers15030729
Shulaev, V. (2006). Metabolomics Technology and Bioinformatics. Briefings in Bioinformatics, 7(2) 128–139. https://doi.org/10.1093/bib/bbl012
Sousa, A. C., Silveira, C., Janeiro, A., Malveiro, S., Oliveira, A. R., Felizardo, M., Nogueira, F., Teixeira, E., Martins, J., & Carmo-Fonseca, M. (2020). Detection of Rare and Novel EGFR Mutations in NSCLC Patients: Implications for Treatment-Decision. Lung Cancer, 139, 35–40. https://doi.org/10.1016/j.lungcan.2019.10.030
St John, E. R., Balog, J., McKenzie, J. S., Rossi, M., Covington, A., Muirhead, L., Bodai, Z., Rosini, F., Speller, A. V. M., Shousha, S., Ramakrishnan, R., Darzi, A., Takats, Z., & Leff, D. R. (2017). Rapid Evaporative Ionisation Mass Spectrometry of Electrosurgical Vapours for the Identification of Breast Pathology: Towards an Intelligent Knife for Breast Cancer Surgery. Breast Cancer Research, 19(1), 59. https://doi.org/10.1186/s13058-017-0845-2
Supplitt, S., Karpinski, P., Sasiadek, M., & Laczmanska, I. (2021). Current Achievements and Applications of Transcriptomics in Personalized Cancer Medicine. International Journal of Molecular Sciences, 22(3), 1422. https://doi.org/10.3390/ijms22031422
T2Biosystems. (n.d.) T2Candidia Panel: Faster Targeted Therapy Leads to Reduced Costs and Improved Outcomes. https://www.t2biosystems.com/products-technology/t2candida-panel/
Tam, V., Patel, N., Turcotte, M., Bossé, Y., Paré, G., & Meyre, D. (2019). Benefits and Limitations of Genome-wide Association Studies. Nature Reviews. Genetics, 20(8), 467–484. https://doi.org/10.1038/s41576-019-0127-1
Temprosa, M., Moore, S. C., Zanetti, K. A., Appel, N., Ruggieri, D., Mazzilli, K. M., Chen, K. L., Kelly, R. S., Lasky-Su, J. A., Loftfield, E., McClain, K., Park, B., Trijsburg, L., Zeleznik, O. A., & Mathé, E. A. (2022). COMETS Analytics: An Online Tool for Analyzing and Meta-Analyzing Metabolomics Data in Large Research Consortia. American Journal of Epidemiology, 191(1), 147–158. https://doi.org/10.1093/aje/kwab120
Tene, O., & Polonetsky, J. (2012, February). Privacy in the Age of Big Data. Stanford Law Review. https://www.stanfordlawreview.org/online/privacy-paradox-privacy-and-big-data/
The DataLad Handbook. (2023). Scaling Up: Managing 80TB and 15 Million Files from the HCP release. https://handbook.datalad.org/en/latest/usecases/HCP_dataset.html
Turk, A. A., & Wisinski, K. B. (2018). PARP Inhibitors in Breast Cancer: Bringing Synthetic Lethality to the Bedside. Cancer, 124(12), 2498–2506. https://doi.org/10.1002/cncr.31307
Tutt, A., Robson, M., Garber, J. E., Domchek, S. M., Audeh, M. W., Weitzel, J. N., Friedlander, M., Arun, B., Loman, N., Schmutzler, R. K., Wardley, A., Mitchell, G., Earl, H., Wickens, M., & Carmichael, J. (2010). Oral Poly(ADP-Ribose) Polymerase Inhibitor Olaparib in Patients with BRCA1 Or BRCA2 Mutations and Advanced Breast Cancer: A Proof-of-Concept Trial. Lancet, 376(9737), 235–244. https://doi.org/10.1016/S0140-6736(10)60892-6
Tzafetas M., Mitra A., Paraskevaidi M., Bodai Z., Kalliala I., Bowden S., Lathouras K., Rosini F., Szasz M., Savage A., Manoli E., Balog J., McKenzie J., Lyons D., Bennett P., MacIntyre D., Ghaem-Maghami S., Takats Z., Kyrgiou M. (2020). The Intelligent Knife (iKnife) and its Intraoperative Diagnostic Advantage for the Treatment of Cervical Disease, Proceedings of the National Academy of Sciences, 117 (13) 7338-7346. https://doi.org/10.1073/pnas.1916960117
van der Hooft, J. J. J., Wandy, J., Barrett, M. P., Burgess, K. E. V., & Rogers, S. (2016). Topic Modeling for Untargeted Substructure Exploration in Metabolomics. Proceedings of the National Academy of Sciences, 113(48), 13738–13743. https://doi.org/10.1073/pnas.1608041113
Wolf-Yadlin, A., Sevecka, M., & MacBeath, G. (2009). Dissecting Protein Function and Signaling Using Protein Microarrays. Current Opinion in Chemical Biology, 13(4), 398–405. https://doi.org/10.1016/j.cbpa.2009.06.027
World Health Organization. (2023, November 21). Antimicrobial Resistance. https://www.who.int/news-room/fact-sheets/detail/antimicrobial-resistance
Comments